Introducing jsluice: A Technical Deep-Dive for JavaScript Gold (Part 2)
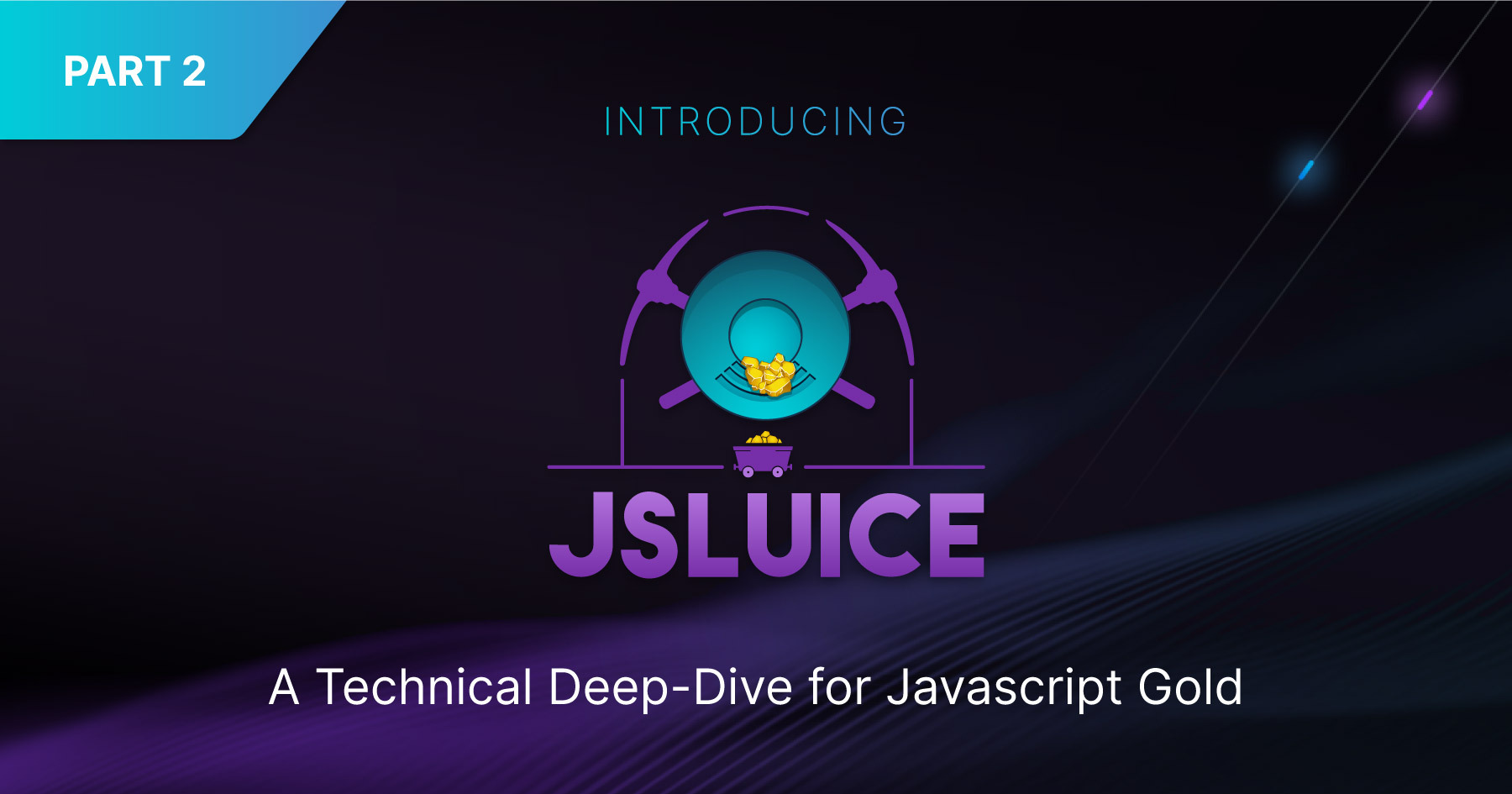
A sluice box is a box lined with riffles or ridges. When you put a sluice box in flowing water that contains little bits of gold, the heavy gold gets stuck in the riffles for you to easily collect, without having to manually sift through tons of dirt and silt.
This is what jsluice
attempts to do for JavaScript - run megabytes of mostly junk though it and get just the interesting bits spat back out at you. There are four modes in jsluice
: urls
, secrets
, tree
, and query
. jsluice
accepts a list of files either as command line arguments or one per line fed into its stdin
. This means you can either run something like this:
jsluice urls fetch.js
Or like this:
find . -name '*.js' | jsluice urls
URLs
Let's go back to that slightly more complicated fetch example we used before and see how the urls mode deals with it:
fetch('/api/v2/guestbook', { method: "POST", headers: { "Content-Type": "application/json" }, body: JSON.stringify({msg: "..."}) })
I've saved that example to a file called fetch.js. jsluice
outputs the JSONL format, i.e. one JSON object per line, so I've piped it to jq to make things a little easier to read:
▶ jsluice urls fetch.js | jq { "url": "/api/v2/guestbook", "queryParams": [], "bodyParams": [], "method": "POST", "headers": { "Content-Type": "application/json" }, "contentType": "application/json", "type": "fetch" }
So jsluice
managed to extract the path, the HTTP method, and the headers. It also labelled the 'type' as 'fetch', so we know where it was extracted from. It didn't extract the body of the request in this case, but nobody's perfect. You could probably make it extract the body too with a bit of work, but in my analysis of several gigabytes of JavaScript gathered from around the web, I found that in most cases the body field is populated with just a variable that we can't easily know the value of anyway.
Let's look at another, slightly more challenging example: XMLHttpRequest
:
function callAPI(method, callback){ var xhr = new XMLHttpRequest(); xhr.onreadystatechange = callback; xhr.open('GET', '/api/' + method + '?format=json', true); xhr.setRequestHeader('Accept', 'application/json'); if (window.env != 'prod'){ xhr.setRequestHeader('X-Env', 'staging') } xhr.send(); }
The problem with code that uses XMLHttpRequest
, for us at least, is that the data we want is spread out between different method-calls. The HTTP method and path are in the call to open, and headers are added using the setRequestHeader
method. One of the calls to add a request header is inside a conditional, further complicating things.
Let's see how jsluice
does:
▶ jsluice urls xhr.js | jq { "url": "/api/EXPR?format=json", "queryParams": [ "format" ], "bodyParams": [], "method": "GET", "headers": { "Accept": "application/json", "X-Env": "staging" }, "type": "XMLHttpRequest.open" }
We managed to extract the path complete with query string, the HTTP method, and the headers even though one of those headers was inside a conditional. To extract the headers, jsluice
is doing something that would be difficult without a syntax tree. The flow looks something like:
- Look for
.open()
calls with at least two arguments - Check the first argument is a valid HTTP method
- Climb the syntax tree to find the containing scope – usually a function definition
- Look for calls to
.setRequestHeader()
only within that scope, on an object of the same name
Neat!
The path it extracted looks a bit funky though because it has EXPR
in the middle of it. The path had a variable called method
concatenated to the end of it, and then a query string concatenated to that:
xhr.open('GET', '/api/' + method + '?format=json', true);
This is exactly the kind of scenario where regular expressions can falter. They might miss the path entirely, only capture the first part, only capture the query string, or maybe just include the quotes and plus signs in their output. None of these options are great. If we were doing dynamic analysis with a real JavaScript engine we could get the value of the method variable, but only if the function was executed.
The static analysis performed by jsluice
collapses these concatenations, replacing any expression with the EXPR
string. This isn't perfect either, and occasionally produces not-so-useful results. However, the result is usually able to be parsed by a URL parser and makes it clear which part of the URL is variable. You might want to use this part of the URL as a place to inject items from a word list.
If you're not happy with EXPR
being the replacement string, you can change it with the --pattern
command-line flag, or if you're using the jsluice
package directly you can set jsluice.ExpressionPlaceholder
to something else. Perhaps the string 'FUZZ' would be a good choice if you're planning on passing the URLs to ffuf.
jsluice
can find URLs, paths, and other request data used in:
- Assignments to
document.location, val.href, val.src, etc
- Calls to
location.replace, window.open, and fetch
- Uses of
XMLHttpRequest
- Calls to jQuery's
$.get, $.post, and $.ajax
- Any other string literal that contains something that looks like a URL
You will sometimes get duplicate matches from that last one, but there's an option, --ignore-
strings
, to disable the feature if you find that to be a problem.
Secrets
URLs and paths aren't the only gold to be found in JavaScript; sometimes there are secrets too. One of the most damaging things we've come across are AWS access keys and their associated secrets. Here's an example object that contains an example key and secret:
var config = { bucket: "examplebucket", awsKey: "AKIAIOSFODNN7EXAMPLE", awsSecret: "wJalrXUtnFEMI/K7MDENG/bPxRfiCYEXAMPLEKEY", server: "someserver.example.com" }
AWS access keys have a nice property that they have a fixed set of prefixes: AKIA, ASIA, AGPA and so on. You can see a full list on this page if you're interested. That makes them easy to write regular expressions for, so why bother adding a feature to jsluice
to extract them?
The first reason is that by using the syntax tree to extract string literals, we don't have to deal with the different kinds of quotes, and that makes writing regular expressions for them easier and more reliable. The second reason is context.
An AWS key by itself can be somewhat interesting, but it's only really damaging if paired with an associated secret. Unlike the key, the secret does not have a common prefix: it's just a block of Base64-encoded data. You can write a regular expression for that, but you will inadvertently match all sorts of other things, and your signal-to-noise ratio will be terrible. Here's what jsluice
's secrets mode does with the above example:
▶ jsluice secrets awskey.js | jq { "kind": "AWSAccessKey", "data": { "key": "AKIAIOSFODNN7EXAMPLE", "secret": "wJalrXUtnFEMI/K7MDENG/bPxRfiCYEXAMPLEKEY" }, "filename": "awskey.js", "severity": "high", "context": { "awsKey": "AKIAIOSFODNN7EXAMPLE", "awsSecret": "wJalrXUtnFEMI/K7MDENG/bPxRfiCYEXAMPLEKEY", "bucket": "examplebucket", "server": "someserver.example.com" } }
There are a couple of important things to note here. The first of which is that both the key and the secret were extracted and put into the data field with predictable key names. If you wanted to pass this data off to another stage of your automation that checks the validity of the key and secret, you can do that!
Secondly, the entire object in which the key and secret were found is included in the context field. There's often relevant information stored alongside credentials; information that can be of great help to a human reviewing these findings. In this example, the AWS key and secret might be for writing to an S3 bucket called examplebucket
, which might serve its content through someserver.example.com
.
jsluice
has built-in detection of keys and secrets for AWS, GCP, and GitHub. There are more secret types to be found than I care to count though, which is why you can also provide your own custom patterns for matching secrets.
Custom Secret Patterns
The jsluice
command-line tool lets you provide a JSON file using the --patterns
or the -p
flag that defines a list of user-defined patterns for matching secrets. Here's a small example patterns file:
[ { "name": "base64", "value": "(eyJ|YTo|Tzo|PD[89]|rO0)[%a-zA-Z0-9+/]+={0,2}", "severity": "low" }, { "name": "genericSecret", "key": "(secret|private|key)", "value": "[%a-zA-Z0-9+/]+" } ]
Each pattern has a name that is used in the kind
field in the tool's output. There are two additional fields: key
and value
. The value
field contains a Go-format regular expression that will be run against all string literals in the JavaScript source code. The quotes will be stripped off, so you don't have to worry about them. The key
field contains a regular expression that will be run against the key names in JavaScript objects. If you specify both fields, both regular expressions will need to match for a result to be returned.
The severity
field lets you categorize your patterns for later prioritization. You probably care more about finding an API key and secret than some Base64-encoded JSON after all.
Here's some, admittedly silly, example code for us to try the above patterns on:
function getConfig(){ let config = { randomStr: "abc123xyz256", secret: "I quite like PHP", } return "eyJsb2wiOiAic29tZSBKU09OISIsICJjb3VudCI6IDEyM30K" }
When we run jsluice
in the secrets mode and provide the patterns file, we get this:
▶ jsluice secrets -p patterns.json b64.js | jq { "kind": "base64", "data": { "match": "eyJsb2wiOiAic29tZSBKU09OISIsICJjb3VudCI6IDEyM30K" }, "filename": "b64.js", "severity": "low", "context": null } { "kind": "genericSecret", "data": { "key": "secret", "value": "I quite like PHP" }, "filename": "b64.js", "severity": "info", "context": { "randomStr": "abc123xyz256", "secret": "I quite like PHP" } }
Our base64
pattern seemed to work fine, but the genericSecret
pattern matched a different kind of secret to the kind we were really hoping for. That's because the regular expression matched part of the value. If you want to stop this kind of thing from happening, you can add anchors to the regular expression. So, this:
[%a-zA-Z0-9+/]+
becomes this:
^[%a-zA-Z0-9+/]+$
And will now match only if the entire value conforms to the regular expression.
Matching Objects
Earlier we used an example of an AWS key and a secret that were in the same object. There are likely to be other situations where you want to match against more than one thing in an object, so the custom patterns support that too! One thing we've come across a few times that are occasionally interesting, are configuration objects for Firebase. They look distinctive; something like this:
let fbConfig = { apiKey: "AIzaSyB47WKzDu9kkmFAsAYFlagkuJxdEXAMPLE", authDomain: "someauthdomain.firebaseapp.com", projectId: "someprojectid", storageBucket: "somebucketthatisnotthere.appspot.com", messagingSenderId: "586572527435", appId: "1:588572526435:web:14c624659103dc3e74b755" }
The object
field in a pattern can be provided as a list of patterns to match against object keys and/or values. If we wanted to match objects like the one above, we might use a pattern like this one:
{ "name": "firebaseConfig", "severity": "medium", "object": [ {"key": "apiKey", "value": "^AIza.+"}, {"key": "authDomain"}, {"key": "projectId"}, {"key": "storageBucket"} ] }
You could add more regular expressions for the values, and make them more specific if you like, but this would be a good starting point.
jsluice
will provide the entire object that was matched in the data
field. The context
field will be set to null
because there's no further context to provide:
▶ jsluice secrets -p patterns.json firebase.js | jq { "kind": "firebaseConfig", "data": { "apiKey": "AIzaSyB47WKzDu9kkmFAsAYFlagkuJxdEXAMPLE", "appId": "1:588572526435:web:14c624659103dc3e74b755", "authDomain": "someauthdomain.firebaseapp.com", "messagingSenderId": "586572527435", "projectId": "someprojectid", "storageBucket": "somebucketthatisnotthere.appspot.com" }, "filename": "firebase.js", "severity": "medium", "context": null }
That's everything you can do with custom patterns. If there's more functionality in this area you'd like to see, let us know! If you want to do anything more complicated in the meantime, you can always dig into the code and write your own matchers with the full power of Go and Tree-sitter at your fingertips.
Trees and Queries
We've already seen jsluice's
tree mode in action, but here's a refresher: it prints a textual representation of the syntax tree for any JavaScript file, like this:
▶ cat hello.js console.log("Hello, world!") ▶ jsluice tree hello.js hello.js: program expression_statement call_expression function: member_expression object: identifier (console) property: property_identifier (log) arguments: arguments string ("Hello, world!")
Now, this is interesting for sure, at least if you're the kind of person who likes syntax trees. It is useful if you want to use jsluice's
other mode though: the query mode.
The query mode lets you run raw Tree-sitter queries against JavaScript files. Now, the Tree-sitter query syntax is a little tricky, and there's some quirks you need to be aware of, but it can be useful for doing analysis on a whole bunch of JavaScript files. We won't cover the full syntax here, but we will look at a few examples to give you a flavor of what's possible. Let's run some queries on the XMLHttpRequest
example code from earlier in this post.
First up, probably just about the simplest thing you could do is extract all the string literals:
▶ jsluice query -q '(string) @match' xhr.js "GET" "/api/" "?format=json" "Accept" "application/json" "prod" "X-Env" "staging"
JSON is the default output format, so jsluice
parsed the strings found in the JavaScript and then re-encoded them using JSON rules. This means that escape sequences like \x20
that are valid in JavaScript but not JSON are interpreted correctly:
▶ cat escapes.js let str = 'Hello,\x20World!' ▶ jsluice query -q '(string) @match' escapes.js "Hello, World!"
If you want the raw data instead of the parsed version, you can use the --raw-output
flag:
▶ jsluice query -q '(string) @match' escapes.js --raw-output 'Hello,\x20World!'
Because jsluice
also understands JavaScript objects, arrays and so on, one of the coolest things you can do with query mode is extract objects from JavaScript and have them converted to valid JSON, ready for further processing and tweaking using tools like jq or gron.
You can take an object like this one:
const config = { stage: false, server: "example.com", ttl: 3600, dns: ["1.1.1.1", "8.8.8.8"], paths: { "home": "/", "blog": "/blog" } }
Turn it into JSON, and then extract just the bits you want using jq:
▶ jsluice query -q '(object) @match' object.js | jq -r 'try .dns[]' 1.1.1.1 8.8.8.8
There's a bunch more you can do with query mode, but that is, as they say, an exercise left for the reader!
Packages
The jsluice
command-line tool can do quite a lot, but if you want to integrate jsluice's
capabilities into your own code, and even extend those capabilities, you might be pleased to hear that the command-line tool is built on top of the jsluice
Go package. This blog post focused almost entirely on the command-line tool as it's the way most people are likely to use jsluice
, but as a parting gift, here's a tiny example program using the jsluice
package.
package main import ( "encoding/json" "fmt" "github.com/bishopfox/jsluice" ) func main() { analyzer := jsluice.NewAnalyzer([]byte(` document.location = "/login?redirect=" + url `)) for _, url := range analyzer.GetURLs() { j, err := json.MarshalIndent(url, "", " ") if err != nil { continue } fmt.Printf("%s\n", j) } }
Thanks for reading this far and let us know what you do with jsluice
. To get started, head over to the GitHub Repository. Happy mining!
Subscribe to our blog and advisories
Be first to learn about latest tools, advisories, and findings.
Thank You! You have been subscribed.