Meet Eyeballer: An AI-powered, Open Source Tool for Assessing External Perimeters
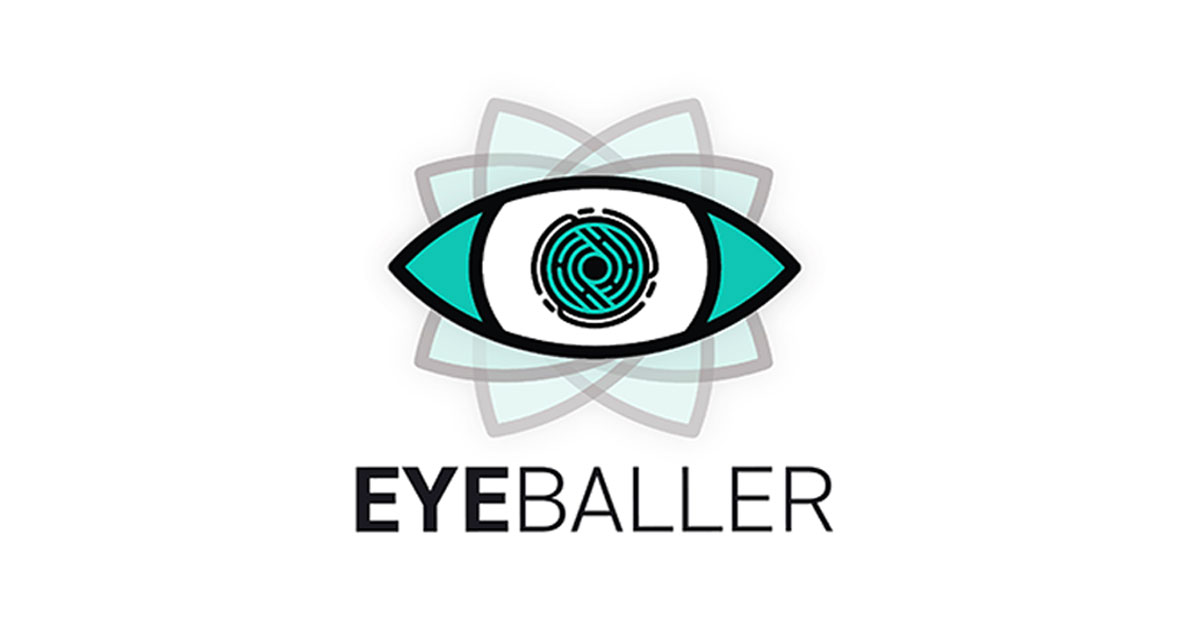
Eyeballer is an AI-powered, open source tool designed to help penetration testers assess large-scale external perimeters.
About Eyeballer
Eyeballer is meant for large-scope network penetration tests where you need to find "interesting" targets from a huge set of web-based hosts. Go ahead and use your favorite screenshotting tool like normal (EyeWitness or GoWitness) and then run them through Eyeballer to tell you what's likely to contain vulnerabilities, and what isn't.
Eyeballer won the 2019 CyberSecurity Breakthrough Award for Web Filtering and Content Evasion. Get the Eyeballer eBook here.
Install Instructions
Go to https://github.com/bishopfox/eyeballer for complete tooling.
Eyeballer uses TF.keras on Tensorflow 2.0. This is (as of this moment) still in "beta". So the pip requirement for it looks a bit weird. It'll also probably conflict with an existing TensorFlow installation if you've got the regular 1.0 version installed. So, heads-up there. But 2.0 should be out of beta and official "soon" according to Google, so this problem ought to solve itself in short order.
Download required packages on pip:
sudo pip3 install -r requirements.txt
Or if you want GPU support:
sudo pip3 install -r requirement-gpu.txt
NOTE: Setting up a GPU for use with TensorFlow is way beyond the scope of this README. There's hardware compatibility to consider, drivers to install... There's a lot. So you're just going to have to figure this part out on your own if you want a GPU. But at least from a Python package perspective, the above requirements file has you covered.
Training Data You can find our training data here:
https://www.dropbox.com/sh/7aouywaid7xptpq/AAD_-I4hAHrDeiosDAQksnBma?dl=1
Pretty soon, we're going to add this as a TensorFlow DataSet, so you don't need to download this separately like this. It'll also let us version the data a bit better. But for now, just deal with it. There's two things you need from the training data:
images/
folder, containing all the screenshots (resized down to 224x140. We'll have the full-size images up soon)labels.csv
that has all the labelsbishop-fox-pretrained-v1.h5
A pretrained weights file you can use right out of the box without training.
Copy all three into the root of the Eyeballer code tree.
Predicting Labels
To eyeball some screenshots, just run the "predict" mode:
eyeballer.py --weights YOUR_WEIGHTS.h5 predict YOUR_FILE.png Or for a whole directory of files:
eyeballer.py --weights YOUR_WEIGHTS.h5 predict PATH_TO/YOUR_FILES/
Eyeballer will spit the results back to you in human readable format (a results.html file so you can browse it easily) and machine readable format (a results.csv file).
Training
To train a new model, run:
eyeballer.py train
You'll want a machine with a good GPU for this to run in a reasonable amount of time. Setting that up is outside the scope of this readme, however.
This will output a new model file (weights.h5 by default).
Evaluation
You just trained a new model, cool! Let's see how well it performs against some images it's never seen before, across a variety of metrics:
eyeballer.py --weights YOUR_WEIGHTS.h5 evaluate
The output will describe the model's accuracy in both recall and precision for each of the program's labels. (Including "none of the above" as a pseudo-label)
Subscribe to our blog and advisories
Be first to learn about latest tools, advisories, and findings.
Thank You! You have been subscribed.