Cyber Mirage: How AI is Shaping the Future of Social Engineering
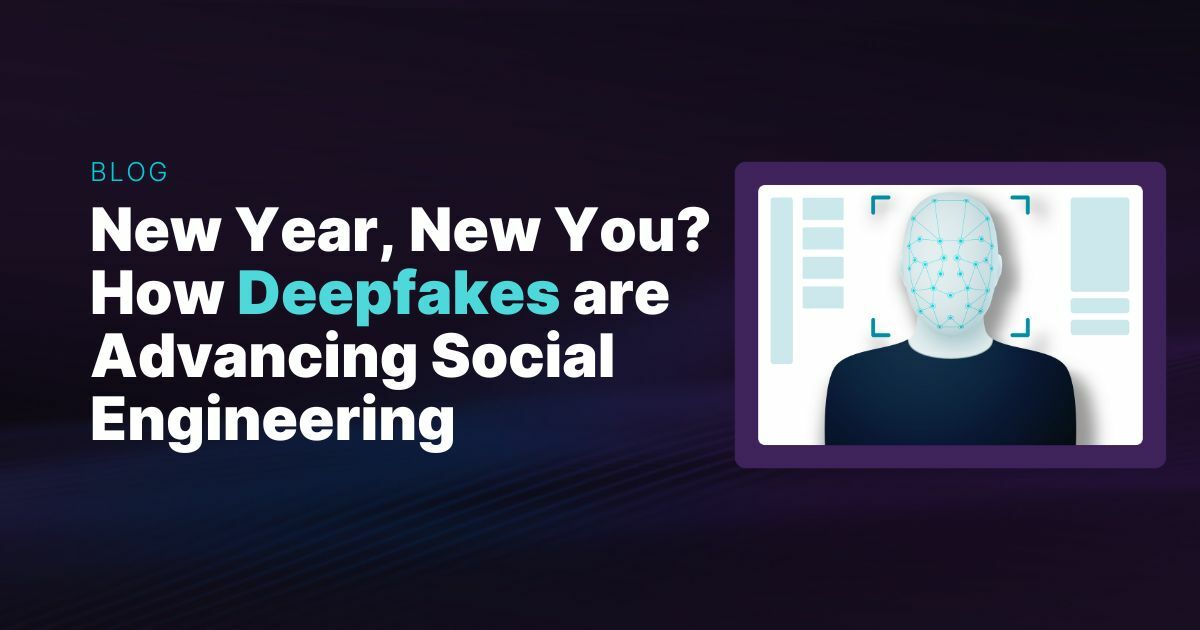
“As a Red Teamer, I’ve always believed the best defense is a great offense. And in this world, that means having a clear understanding of the tactics, techniques, and procedures, because you can’t defend against a threat without first understanding the threat and how it really works.” – Brandon Kovacs, Senior Security Consultant
There is no doubt about it: AI is shaping the future of social engineering. AI has given rise to a new class of cyberattacks that enable attackers to create hyper-realistic deepfake video and voice impersonations with the intent of manipulating or deceiving their victims into divulging sensitive or take actions they otherwise wouldn’t take.
We saw this play out in the news earlier this year when a finance professional at a global corporation in Hong Kong was deceived into transferring $25 million to scammers, who leveraged deepfake and voice-cloning technology to impersonate the company's chief financial officer on a video conference call.
In light of this alarming incident and so many others, Senior Security Consultant Brandon Kovacs explored how deepfakes and voice cloning are created and how companies can defend their organizations against these advanced attacks in a highly attended webcast.
How AI-Powered Attacks are Created: Understanding the Terminology
Deepfakes and voice impersonations are made by training on data collected from public sources, including social media posts, podcasts, interviews, and earnings calls.
Let’s drill down into what that means. Here are a few terms to lay the foundation of understanding.
- Dataset – A collection of data used to train the model. Typically expressed as a set of inputs and desired outputs.
- Model – A type of algorithm that relies on data to recognize and make predictions on new, unseen data.
- Training – The process of teaching a model, through trial and error, to recognize patterns within a dataset.
- Inferencing – The process of using a trained model to make predictions and conclusions on new, unseen data. The phase most people are familiar with.
Training Vocal Clones
All models are trained by going through a series of steps, and this process is adapted depending on whether you’re creating a deepfake video or voice clone model.
Data Collection
The process begins with collecting data, whether text, photos, videos, audio files, or documents.
Using retrieval voice conversion (RVC), you can train your own vocal models with WAV audio files as the training data. RVC models can then be inferred to perform audio-to-audio conversion, or vocal cloning. Essentially, you train a model with a source audio dataset and are then able to manipulate your voice’s tone and pitch to match the subject’s voice with remarkable accuracy.
Data Preparation
Next, you must prepare the data by cleaning and formatting.
- Clean – Cleaning the data involves removing the audio files of any delays, background noise, or sections of muffled speech.
- Splice – Splice the files by cutting them into chunks of 10 seconds or less.
- Convert – Next, convert and export the files into WAV format to ensure high-quality lossless audio. These steps can be performed using free software, such as Audacity, or paid software, such as Adobe Audition.
- Transcribe – Text transcription of the audio files is the final step; it is optional but can greatly enhance your results.
Training
Once the data has been cleaned and prepared, you are ready to train the data.
The software takes the audio files you’ve uploaded, analyzes it, and recognizes patterns in the voice. From there, it creates a trained model from that specific individual’s voice which can then be used to infer what someone sounds like - in essence, having the ability to impersonate or replicate an individual’s voice.
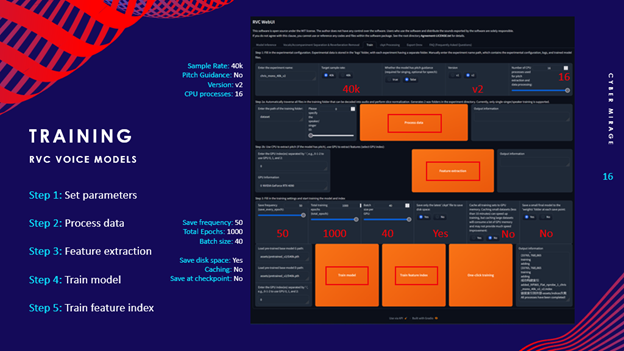
Vocal cloning technology offers a wide range of potential benefits, from creating personalized virtual assistants to improving accessibility for those who cannot speak. However, there are serious ethical and security implications associated with this technology that must be considered.
Training Deepfakes
In the webcast, Brandon gave a live demo of a deepfake model, using an open-source tool, to impersonate his buddy Chris (with his permission, of course). In this section, you’ll see screenshots from the process Brandon completed and a clip of Brandon deepfaking Chris in real time.
To create the deepfake, Brandon used DeepFaceLab, a popular open-source tool for creating deepfake models that is “purportedly responsible for 95% of deepfakes on the internet” according to their GitHub repository. This technology uses advanced machine learning techniques to train a deepfake video model using photos as the training data. Through a process known as “merging,” the face is swapped from the source to the destination.
To create a deepfake, you will go through the same general method as that of vocal cloning - you will have to collect, prepare, and train the data to achieve the intended results. Let’s dive in.
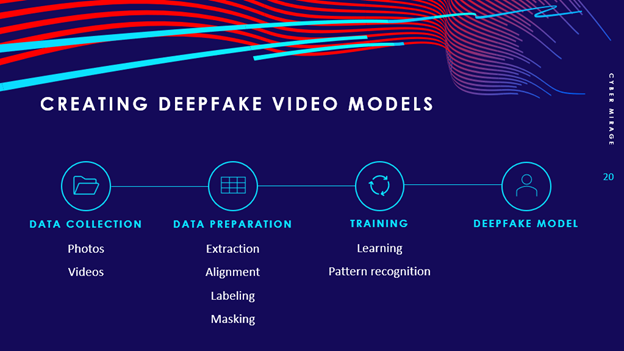
Data Collection
For deepfake videos, you may collect photos or videos. This is best achieved with high-quality videos filmed in a studio but can also be achieved by collecting video from publicly available content. The videos of the subject should have a variety of angles, facial expressions and lighting conditions.
Data Preparation
Data preparation for deepfakes revolves around identifying and defining the source and the destination. The source is the original person or object that is being cloned (i.e. the public figure). The destination is the outcome of the deepfake manipulation (i.e. the individual who is going to impersonate the source) where the sources face will appear on.
Essentially, deepfake technology takes a source (the original content) and transforms it onto the destination (the manipulated or fabricated content). Preparing the data is a lengthy, multi-step process:
- Extraction – DeepFaceLab uses photos as the training data. To prepare the data set for training, you must first extract the frames of any recorded videos for both the source and destination persons. To accomplish this, you can use an open-source tool such as FFmpeg to extract the image frames from the source and destination videos.
- Alignment – DeepFaceLab then examines the image frames to identify the faces and facial landmarks (i.e. the eyes, nose, and mouth).
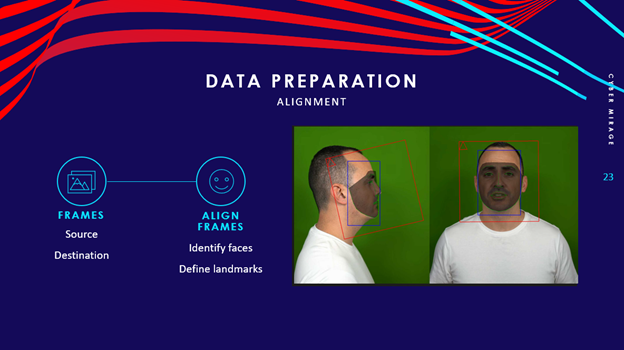
- Labeling – Labeling is a critical step in the process where you manually annotate the image frames to define the edges of the face and any obstructions, such as hats or glasses, for both the source and destination subjects. This should be done to a number of images with a variety of angles and facial expressions.
- Masking – Masking is a complex process that uses the labeled image data to train a XSeg mask model that is capable of recognizing the facial contours for all of the images in the dataset for the source and destination, while ignoring any obstructions. This process can take anywhere from a few days to a few weeks, depending on compute hardware.
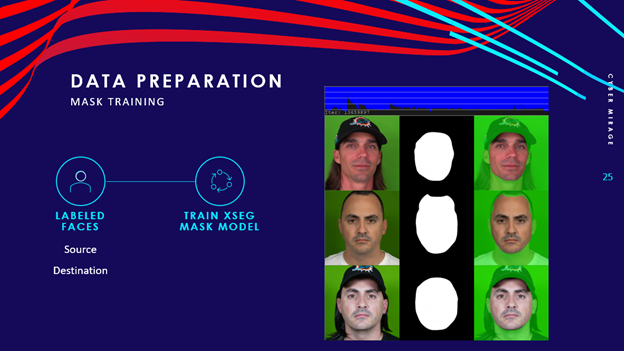
During the masking phase, the model learned to recognize both Brandon and Chris’ faces while ignoring obstructions (such as hats).
Training
After the lengthy process of preparing the data and preparing an Xseg mask model, you can now train the deepfake video model. During the training step, the model is taught to recognize patterns in both the source and destination, learn from it, and make a series of predictions based on what it knows at that point in time.
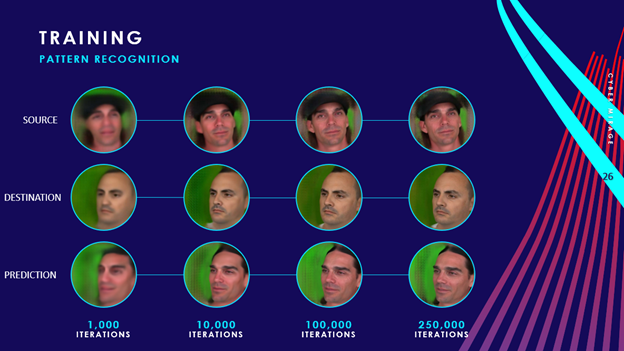
Top row: Predictions of source (Chris)
Middle row: Predictions of destination (Brandon)
Bottom row: Face-swapping prediction between the source and destination
As the number of iterations increases, the images become more lifelike and realistic. Using DeepFaceLive (a separate tool), you can use the model that you created and trained using DeepFaceLab to do a live deepfake.
The following clip is a timelapse showing the evolution of the model as it is being trained. The two far left columns represent the source-to-source prediction, the middle columns represent the destination-to-destination prediction, and the far-right column is the deepfake prediction, swapping Chris and Brandon’s faces.
Deepfaking Chris
Take a look at the final product. Here is the live demo of the final output in the webcast showing the results of the trained model where Brandon was able to deepfake his Chris in real time.
Using Deepfakes and Voice Clones for Offensive Security
Deepfakes and voice clones are a new class of AI-powered cyberattacks with wide-reaching implications for society and business. Here’s how Brandon recommends incorporating this technology into your Red Team exercises to ensure your organization is equipped to defend against these types of threats.
Two examples include:
- Use the vocal clone model during an external breach to impersonate the subject and attempt to perform a password reset against the IT Help Desk.
- Leverage the deepfake video and vocal model to perform a real-time deepfake over an internal video conferencing software during an assumed breach scenario to gain trust and manipulate employees into allowing you to pivot and move laterally.
How to Protect Against Vocal Clones and Deepfakes
The bottom line with this technology is that it will affect everyone. Attackers are more widely using this technology for phishing and vishing scams at large, and the frequency and sophistication of attacks will only increase. It will impact small, medium and large businesses alike, along with vulnerable and elderly individuals.
And if you are a public figure, you are even more at risk of being impersonated. There is a lot of publicly-available content that exists – earnings calls, interviews, podcasts, social media blasts, networking forums, company website – and all of it can be used to train a video model or voice model of you.
With that, we have two practical ideas for operational process improvements to mitigate the risk of fraud in your organization. In a scenario where an individual requests an action to be taken (such as transferring money) – especially while employing fear, uncertainty, or doubt (FUD) – you need to establish caller’s identity to ensure the validity of the request.
Two ways to do that are:
- Calling back the perceived requester using a verified phone number that you look up in the official corporate directory. Remember, attackers can perform caller ID manipulation to make it appear like the impersonated individual is calling.
- Add a layer of two-factor authentication by asking the caller for a previously agreed upon safe word or passcode that only you and that individual know. By establishing a set passcode, you will be able to authenticate the individual making the request.
These are both low- or no-tech approaches that enable you to cut the attacker out of the loop. Even in the face of cutting edge cyberattacks, sometimes old school methods work best.
Conclusion
Cloning technology has several great benefits for society, but as always, threat actors can use something good for nefarious purposes. To stay ahead of the curve, we must continue to assess our security posture, educate our workforce, and remain vigilant in the face of evolving threats.
For more information on social engineering and how best to protect your organization from these attacks, check out a few additional resources:
Subscribe to our blog and advisories
Be first to learn about latest tools, advisories, and findings.
Thank You! You have been subscribed.